On the afternoon of October 9, the Royal Swedish Academy of Sciences decided to award the 2024 Nobel Prize in Chemistry to three scientists. Among them, AI model AlphaFold2, which predicts complex protein structures, became a global focal point. The prize went to 48-year-old Google AI leader Demis Hassabis and 39-year-old John Jumper, highlighting the immense potential of AI in the field of chemistry. This article summarizes some applications of AI in chemical processes, showcasing AI’s contributions to improving industry efficiency and advancing digital transformation.
1. AI Applications in Chemical Synthesis
Automation and real-time reaction monitoring have enabled data-rich experiments, which are essential for addressing the complexities of chemical synthesis such as ethylene carbonate synthesis and polylactic acid synthesis. By combining real-time analysis with machine learning (ML) and artificial intelligence (AI) tools, it becomes possible to accelerate the identification of optimal reaction conditions and facilitate error-free autonomous synthesis.
Most molecule synthesis requires multi-step transformations, balancing material inputs (such as solvents, reagents, and catalysts), reaction parameters (temperature, addition order, and time), and purification strategies. Tackling these multifactorial challenges is akin to navigating a maze with limited resources. Historically, chemists had to rely on past experiences, formulating cautious strategies, and making decisions based on limited data. AI automation has transformed this landscape by significantly improving both the quantity and accuracy of reaction data analysis, enabling better decisions to be made in a shorter timeframe. For instance, high-throughput experimentation (HTE) techniques can rapidly investigate potential reaction conditions, but these techniques often provide analysis yields at fixed time points, missing crucial details related to reaction mechanisms or kinetics.
Figure: Super-efficient liquid chromatography analysis of the Suzuki-Miyaura cross-coupling reaction, showing different peak areas of starting materials and products, as well as common by-products, over time.
Machine learning and AI tools are powerful complements to data-driven workflows in experiments, accelerating the identification of reaction conditions. Predictive models built from high-throughput experimental (HTE) data or literature sources can suggest reaction conditions for previously untested transformations. Moreover, by integrating machine learning optimization algorithms with robotic reaction execution, endpoint sampling, and data extraction, autonomous optimization platforms have been created. These methods can reduce the number of experiments needed to identify ideal conditions, but they tend to simplify experimental results into single quantitative scores, such as yield or stereoselectivity percentages. While these strategies have their advantages, reducing experimental results to a single measurement at a fixed time oversimplifies the inherent complexity of chemical reactions.
Many studies have shown mixed results when using reaction performance data (such as yield) from existing literature. Data is biased towards the most commonly published conditions, often selecting conventional reaction parameters rather than the optimal ones. Worse, the heterogeneity of quantitative measurements and applied conditions makes it difficult to distinguish whether reported yields reflect experimental failures or challenges in product separation. Efforts to systematize synthesis data are emerging but remain in the early stages.
Real-time reaction monitoring offers a key advantage: by using comprehensive kinetic data, predictive models can be trained. These comprehensive data address issues related to data integrity, biases, and oversimplification. By recording the entire reaction profile, differences in reaction performance under different experimental conditions can be captured and interpreted. Furthermore, the complete evolution of reactants can be tracked, allowing the changes in target materials, by-products, and intermediates to be described. These trends provide useful source data for future reaction processes, as they capture potential transformations outside the immediate research focus. Overall, machine learning (ML) methods are well-suited to training models that reflect the full complexity of reactions.
The data science revolution in synthetic chemistry is accelerating, driving an increased demand for rich experimental data. Real-time reaction analysis has already been used to dramatically reduce the time needed to reach target molecules. By further integrating these automated data collection methods with new ML and AI tools, our ability to predict optimal conditions and discover new synthetic pathways will grow exponentially.
2. AI Applications in Chemical Manufacturing Processes
As a key component of process industries, chemical manufacturing involves numerous chemical reactions and material transformations. In industrial operations, AI can now assist engineers and data scientists in carrying out routine tasks. It can help integrate external data sources using natural or computer language and work in conjunction with other solutions. For example, in chemical manufacturing processes, AI can generate large model schemes by training on operational data and may propose action plan suggestions based on existing information, though final decisions still rest with engineers.
AI plays a more active role by automating routine tasks. It follows predefined rules and procedures, reducing human intervention in daily activities. Automation intelligence is commonly seen in robotic processes, such as machines sorting materials or products on a conveyor belt.
In another example, generative AI can assist in coding for tasks requiring the creation of machine learning models or other computer language-based operations. It can also store and retrieve information, acting as a repository for use cases and corresponding normative information, which can be accessed and extracted through natural language prompts. When combined with other technologies, generative AI becomes even more effective. For example, by using Retrieval-Augmented Generation (RAG), chatbots can be added to these databases. Through API calls, engineers can access advanced industrial analysis software and interact with it directly. They might simply ask, "What happened after my shift?" and the RAG-enabled GPT would generate a detailed summary of the events during that time period.
However, the implementation of AI also presents unique challenges. Most importantly, the quality and integrity of data are critical. The effectiveness of AI systems depends on the data they process. Inaccurate or incomplete data can lead to erroneous insights and decisions. Therefore, providing accurate data to these solutions is essential.
Chemical process manufacturing is still in the experimental phase of AI implementation. Companies are learning how and where to harness these solutions' benefits while mitigating potential risks. Since manufacturing processes still require the decision-making skills of engineers, the industry is proceeding with caution. Nevertheless, an increasing number of chemical companies are experimenting with digital transformation, with practical applications like machine learning models and dashboards steadily emerging.
3. AI in "Anomaly Detection" in Chemical Processes
Anomaly detection is often cited as an AI application case. For example, business experts can search for benchmark values in time series data to identify anomalies in expected patterns. These anomalies are presented in an easy-to-understand way, enabling quick responses and decisions. By creating unique markers for ideal batch parameters from contextual data, anomaly detection helps define and detect abnormal situations. In more advanced cases, models developed using self-organizing maps (SOM) can detect both global and local anomalies within multivariate contexts.
At a specialty chemical company, process engineers have recognized the benefits of using this technology for anomaly detection. By processing operational data with advanced industrial analysis software, data scientists have developed models that include soft sensors, anomaly detection scores, and predictive maintenance alerts.
The integration of these machine learning capabilities has led to significant operational improvements at the company. Notably, the batch processing time has been reduced by 10%, equivalent to one fewer batch per day. Additionally, the improvement in operational efficiency has resulted in a 9% reduction in energy consumption.
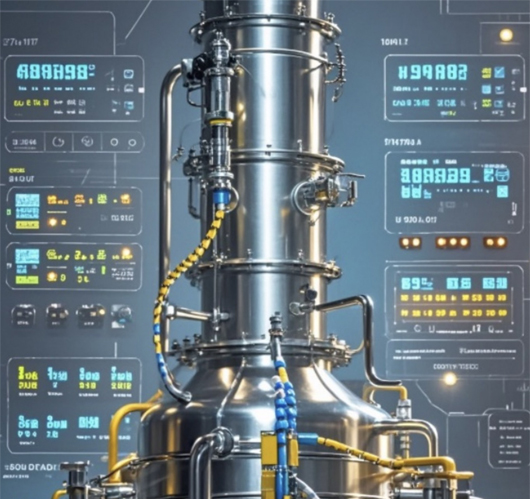
Conclusion: AI is steadily evolving, and technological advances are progressing rapidly. By harnessing large volumes of existing data, engineers can gain instant insights to optimize operations. At the same time, companies are taking many necessary steps to leverage AI for future success.