On the afternoon of October 9, the Royal Swedish Academy of Sciences announced the recipients of the 2024 Nobel Prize in Chemistry. Among the winners, the AI model AlphaFold2, which predicts complex protein structures, became the global focus. Google AI leader Demis Hassabis, 48, and John Jumper, 39, were awarded the Nobel Prize in Chemistry, highlighting the tremendous potential of AI in the field of chemistry. This article summarizes some applications of AI in chemical processes, showcasing its contributions to improving industry efficiency and advancing digital transformation in the sector.
I. Application of AI in Chemical Synthesis
Automation and real-time reaction monitoring have enabled data-rich experiments, which are crucial in addressing the complexity of chemical synthesis. The combination of real-time analysis with machine learning and artificial intelligence tools offers the opportunity to accelerate the determination of optimal reaction conditions and facilitate error-free autonomous synthesis.
Synthesizing most molecules requires multi-step transformations, balancing the input of materials (e.g., solvents, reagents, catalysts), reaction parameters (e.g., temperature, order of addition, time), and purification strategies. Addressing such multi-factor challenges is akin to navigating a maze with limited resources. Historically, chemists had to rely on past experience, formulate cautious strategies, and make decisions based on limited data.AI-driven automation has transformed this landscape, significantly enhancing the volume and accuracy of reaction data analysis, allowing better decisions to be made in less time. For instance, high-throughput experimentation (HTE) can be employed to quickly investigate potential reaction conditions. However, such techniques often only provide analysis yields for fixed reaction times, neglecting critical details related to reaction mechanisms or kinetics (as shown in the figure below).
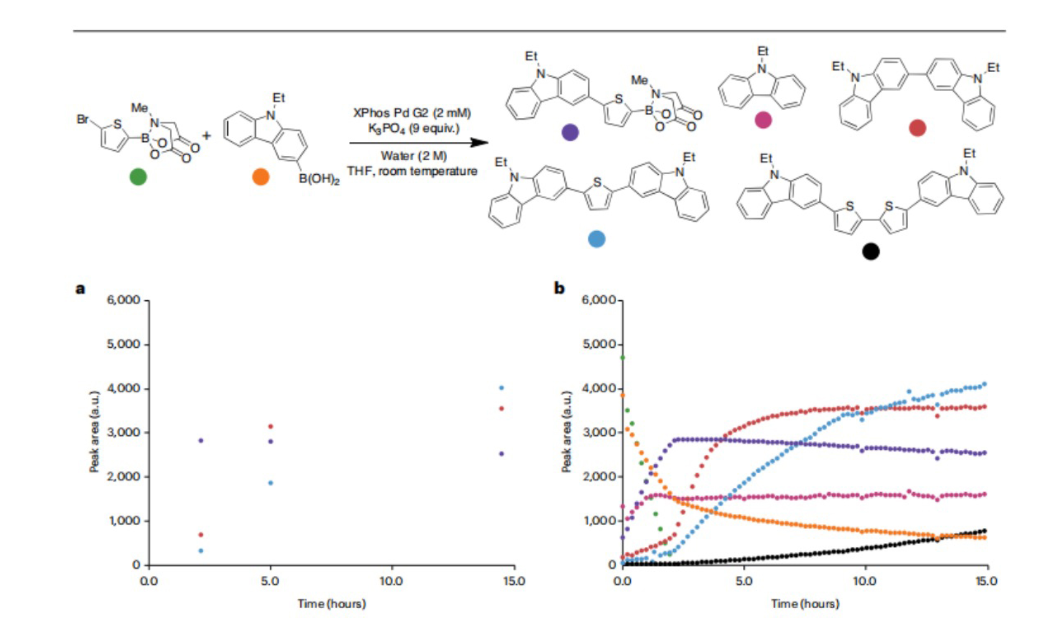
Suzuki – Miycross-coupling by HPLC showed different peak areas over time for the start and product as well as common by-products. a. Limited understanding was obtained when a small number of time points were captured. b. The same transformation is visualised into a complete reaction profile, providing a full view of the reaction immediately. a. U., any unit; XPhos Pd G2, chloride (2 - dicyclohexyl phosphonate-2 ', 4', 6 '- triisopropyl-1,1' - biphenyl) [2 - (2 '- amino-1,1' - biphenyl)] palladium (II); THF, tetrahydrofuran.
Machine learning (ML) and artificial intelligence (AI) tools are powerful complements to experimental data-driven workflows, accelerating the identification of reaction conditions. Predictive models, built from experimental data obtained through high-throughput experimentation (HTE) or literature sources, can propose reaction conditions for executing unknown transformations.Moreover, autonomous optimization platforms have been developed by integrating ML optimization algorithms with robotic reaction execution, endpoint sampling, and data extraction. These methods reduce the number of experiments needed to identify ideal conditions.However, both approaches simplify experimental results into single quantitative metrics, such as yield or stereoselectivity percentages. While these strategies have certain advantages, reducing experimental outcomes to single measurements at fixed time points obscures the inherent complexity of chemical reactions.
Many studies have shown that extracting reaction performance data (yields) from existing literature yields mixed results. The data are often biased toward the most frequently published conditions, leading to the selection of conventional reaction parameters rather than optimal ones.
Worse still, the heterogeneity in quantitative measurements, as well as the conditions or techniques applied, makes it difficult to determine whether the reported yields reflect experimental failure or challenges in product isolation. Efforts to systematize synthetic data are emerging but remain in their early stages.
Real-time reaction monitoring offers a key advantage: comprehensive kinetic data can be used to train predictive models. These complete datasets address issues related to data integrity, bias, and oversimplification.First, by recording the full reaction profile, variations in reaction performance across different researchers’ operations can be captured and interpreted. Second, the entire evolution of reactants is documented, enabling the characterization of target materials, by-products, and intermediates.These trends serve as valuable source data for future reaction studies, as they capture transformations that may fall outside the primary focus of the research. Overall, machine learning (ML) methodologies are particularly well-suited for training models to reflect the intricate patterns of entire reactions.
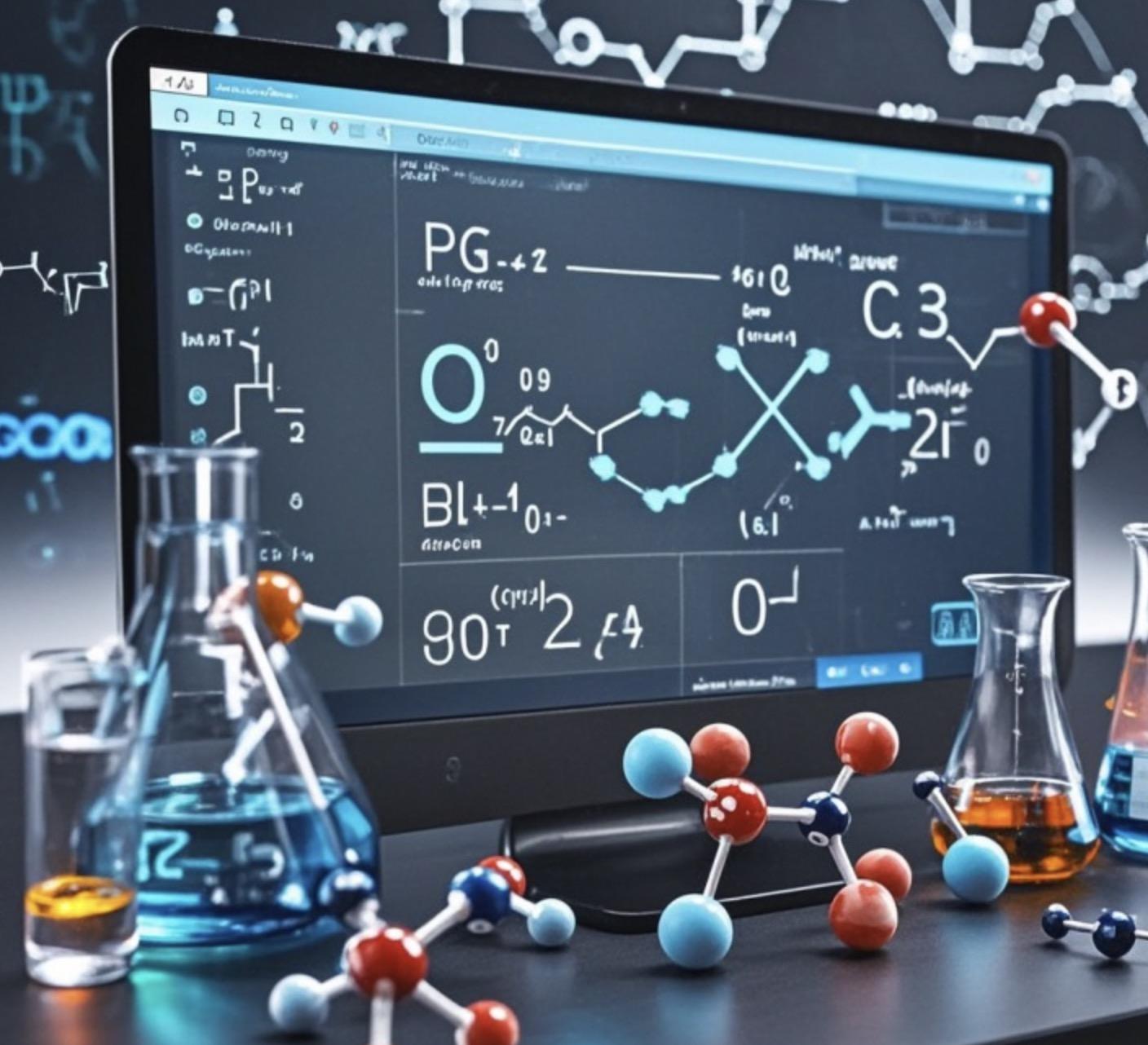
The data science revolution in synthetic chemistry is accelerating, increasing the demand for rich experimental data. Real-time reaction analysis has been utilized to significantly reduce the time required to achieve target molecules. By further combining these automated data collection methods with new machine learning and artificial intelligence tools, we predict that the ability to predict optimal conditions and discover new synthetic pathways will grow exponentially.
II. Application of AI in Chemical Manufacturing Processes
As a crucial part of the process industry, chemical manufacturing equipment involves numerous chemical reactions and material transformations. In industrial operations, AI is already being used to assist engineers and data scientists in performing daily tasks. It can help integrate external data sources, work with other solutions, and interact using either natural or programming languages. For instance, in the production processes of chemical enterprises, AI can generate large model-based solutions through training with operational data, suggesting actions based on existing information. However, the final decision lies in the hands of the engineers.
Here, AI plays a more proactive role by automating daily tasks. It follows predefined rules and procedures, reducing human intervention in routine activities. Automated intelligence is commonly seen in robotic processes, such as machines sorting materials or products on a conveyor belt.
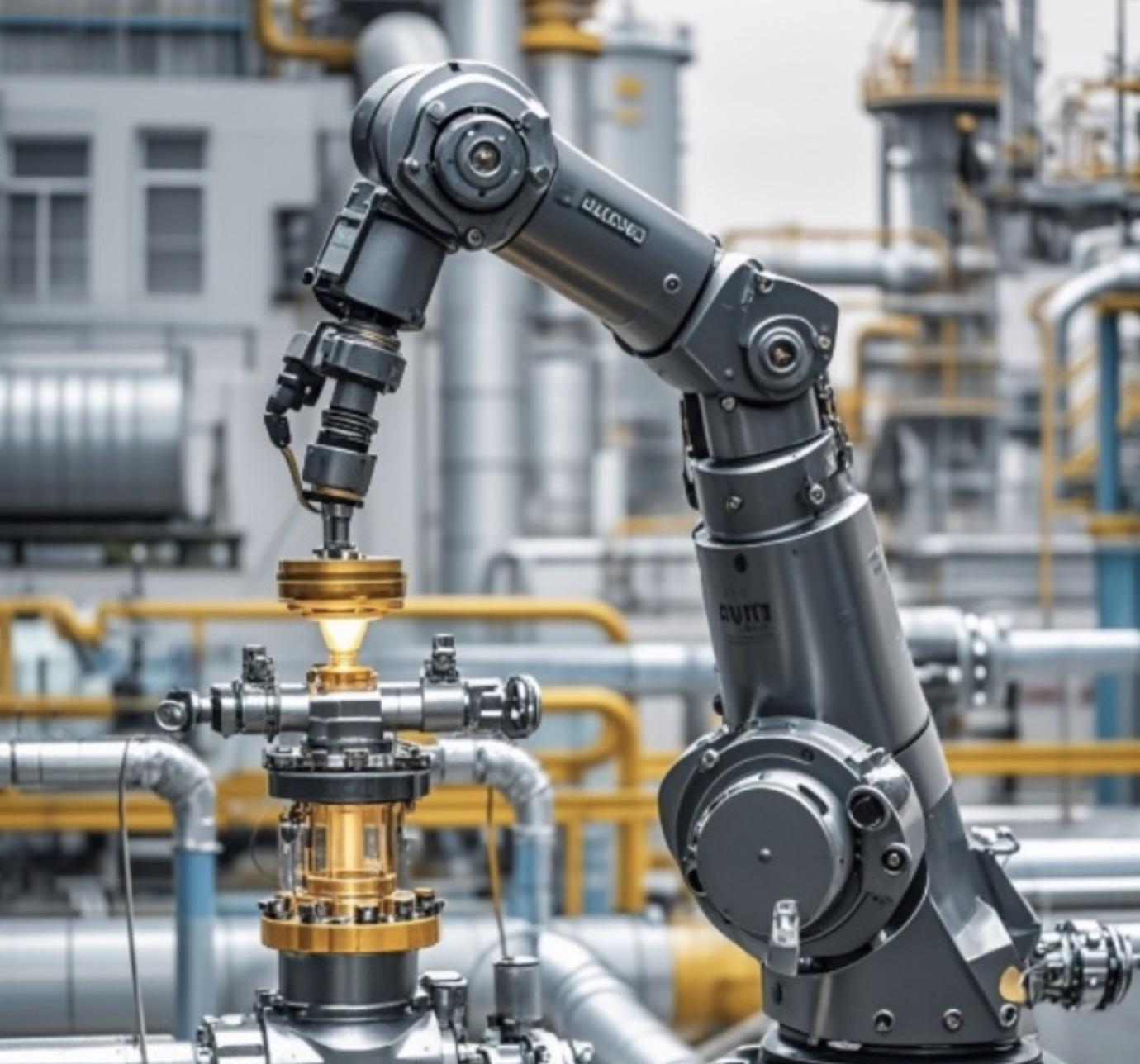
In another example, generative AI can assist in coding tasks for creating machine learning models or other tasks that require computer programming languages. It can also store and retrieve information, making it a useful repository for use cases and corresponding normative information, which can be found and extracted through natural language prompts. The effectiveness is enhanced when generative AI is combined with other technologies. For example, by utilizing Retrieval-Augmented Generation (RAG), chatbots can be added to these databases. Through API calls, engineers can access advanced industrial analytics software and interact with it directly. They can simply ask, “What happened after I got to work?” and the GPT enabled with RAG will generate a detailed summary of events during that period.
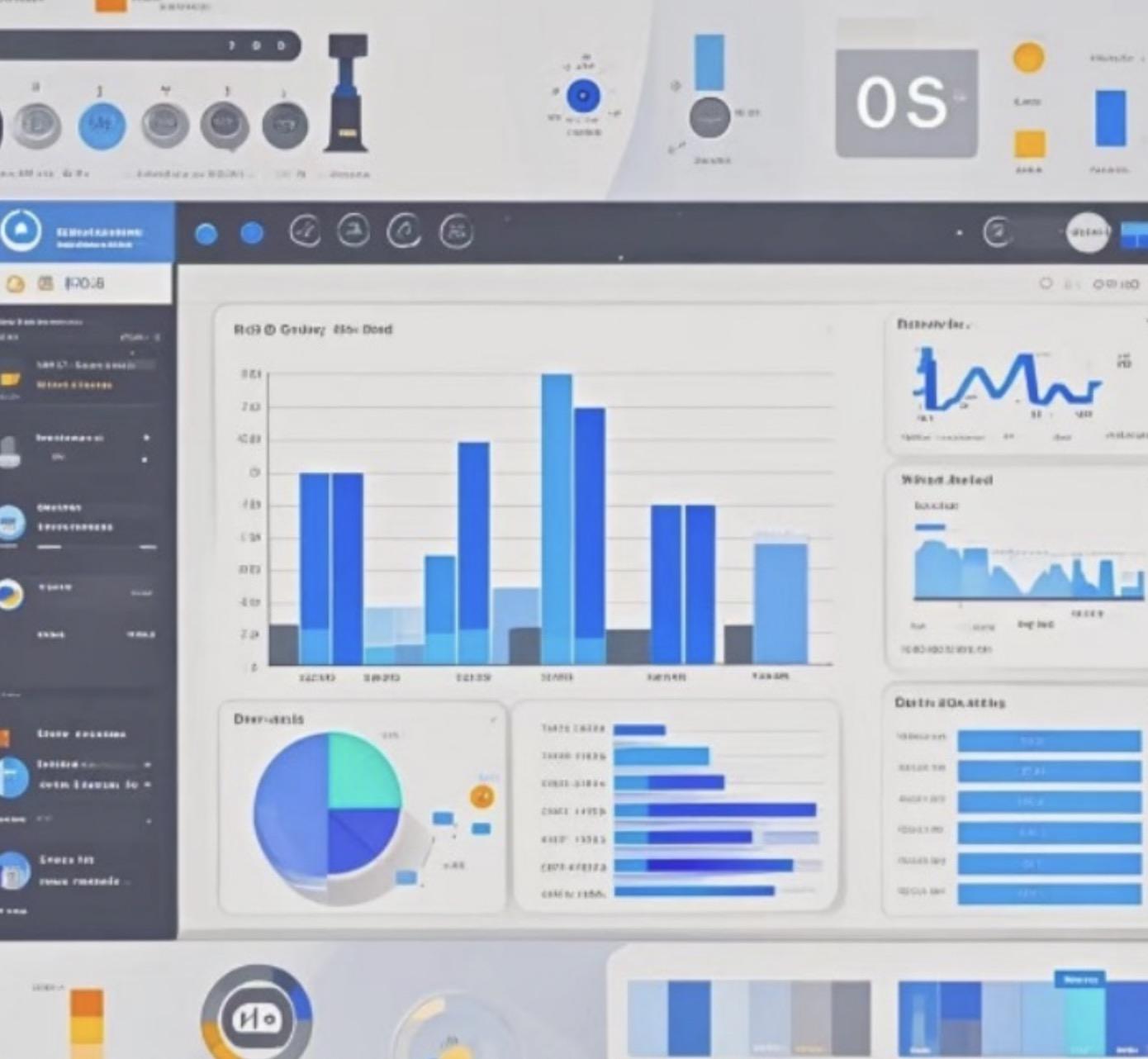
However, the implementation of artificial intelligence also encounters a series of unique challenges. The most important of these is the quality and integrity of the data. The effectiveness of AI systems depends on the data they process. Inaccurate or incomplete data can lead to incorrect insights and decisions. Therefore, it is crucial to provide accurate data for these solutions.
The chemical process manufacturing industry is still in the experimental phase of AI. Companies are learning how and where to leverage the advantages of these solutions over potential risks. Since manufacturing processes still require engineers’ decision-making skills, the industry is proceeding cautiously. Nonetheless, more and more chemical enterprises are attempting digital transformation, and practical applications, such as the creation of machine learning (ML) models and dashboards, are continually emerging.
III. Application of AI in “Anomaly Detection” in Chemical Processes
As an application of artificial intelligence, “anomaly detection” is frequently mentioned. For example, business experts can search for benchmark values in time-series data to identify anomalies in expected patterns. These anomalies are presented in an easily understandable way to enable quick responses and decisions, creating unique markers for ideal batch parameters from contextual data, which helps define and detect anomalies. In more advanced cases, models developed using Self-Organizing Maps (SOM) can detect both global and local anomalies in a multidimensional context.
At a specialty chemicals company, process engineers recognized the benefits of using this technology for anomaly detection. By processing operational data with advanced industrial analytics software, data scientists developed models that include soft sensors, anomaly detection scores, and predictive maintenance alerts.
The integration of these machine learning capabilities led to significant improvements in the company’s operations. Notably, the company reduced its batch processing time by 10%, equivalent to one fewer batch per day. Additionally, improved operational efficiency resulted in a 9% reduction in energy consumption.
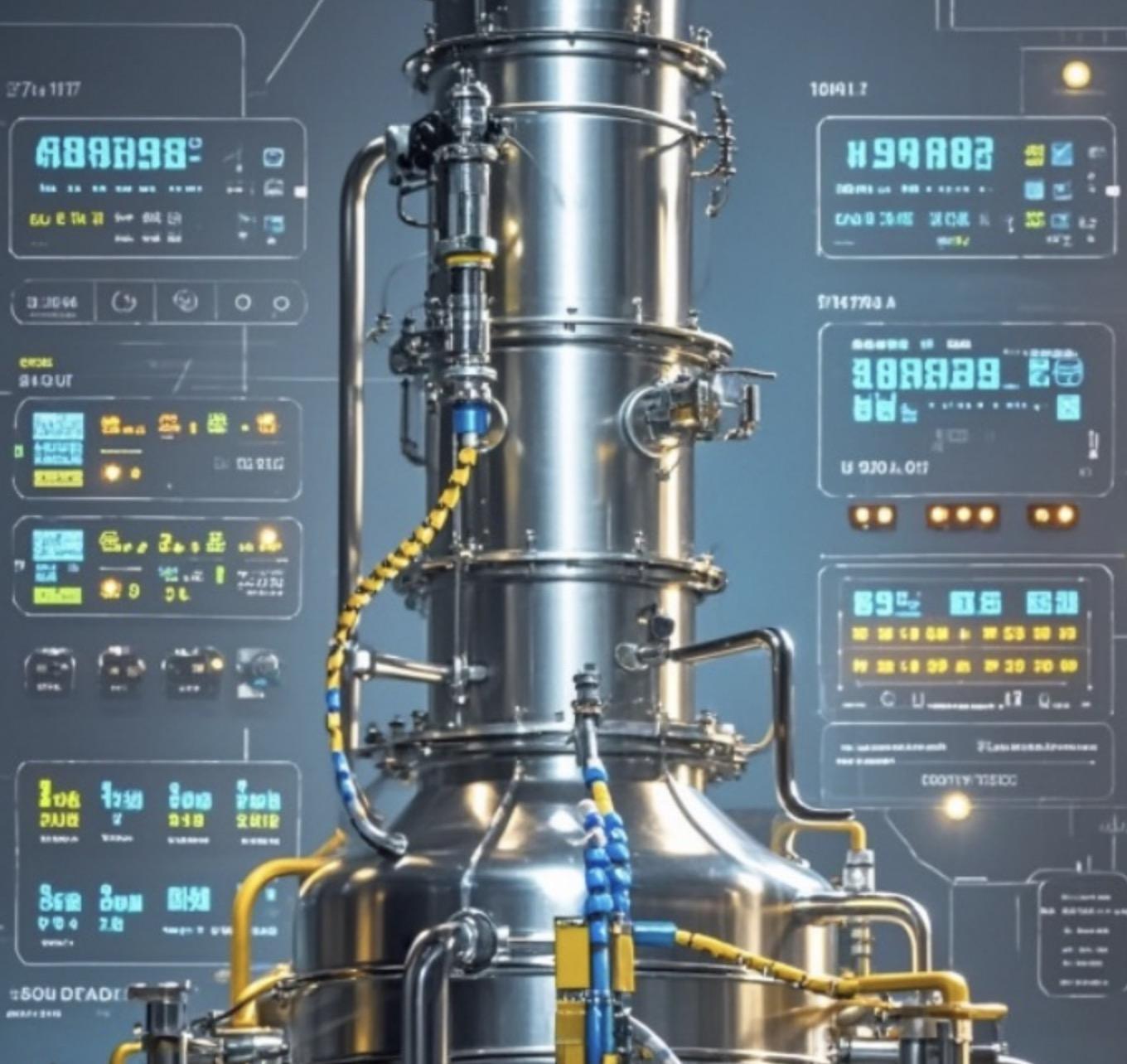
Conclusion:
Artificial intelligence is steadily advancing, with technological progress occurring at an accelerating pace. By leveraging the vast amounts of data available, engineers can gain real-time insights, optimizing operations. At the same time, businesses are taking many necessary steps to harness AI for future success.